Speech by Rob Gruppetta, Head of the Financial Crime Department at the FCA, delivered to the FinTech Innovation in AML and Digital ID regional event, London.
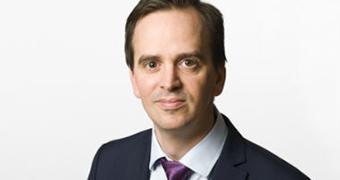
Speaker: Rob Gruppetta, Head of the Financial Crime
Event: FinTech Innovation in AML and Digital ID regional event, London
Delivered: 06 December 2017
Note: this is the speech as drafted and may differ from the delivered version
Highlights
- Criminals must be confident the money cannot be traced back to their crimes; they make huge efforts to launder dirty funds while banks and other financial firms make similar efforts to prevent abuse of their services by criminals.
- Could software be taught human intuition that senses something is not quite right?
- Could machines trawl through a bank’s transactions to detect suspicious activity in real time?
Are you suspicious? This is a key question at the heart of efforts to tackle money laundering: if you work for a bank or other financial institution and have suspicions money laundering is happening, you have a legal duty to speak up. Your suspicion could be based on pure intuition - a sense that something just doesn’t quite add up - but the law nonetheless expects you to act.
Across a huge institution, however, processing millions of transactions per hour, only a tiny share of customers will meet an actual human being. Could software be taught the human intuition that senses something is not quite right? Or can it pick out suspicious behaviour that even humans might not notice? Financial firms are increasingly asking us these questions.
Trade bodies estimate British banks spend £5bn annually combating financial crime, a sum that is £1bn more than Britain spends on prisons.
To enjoy their ill-gotten gains, criminals must be confident the money cannot be traced back to their crimes; they make huge efforts to launder dirty funds. Banks and other financial firms make similar efforts to prevent abuse of their services by criminals. Trade bodies estimate British banks spend £5bn annually combating financial crime, a sum that is £1bn more than Britain spends on prisons.
A big share of this is spent on detecting suspicious activity and reporting it to the authorities; anomalous transactions might be reported by a cashier who smelt a rat or by systems that spot when pre-determined thresholds have been exceeded: dedicated teams then determine whether it truly is suspicious and liaise with the appropriate authorities if it is.
Some have asked whether machine learning and artificial intelligence techniques could help here. Could machines trawl through a bank’s transactions, for example, to detect suspicious activity in real time? Data analytics and machine learning are widely seen as the approaches with the greatest potential to improve current practices, particularly in the field of transaction monitoring.
Better transaction monitoring is not the only way AI can aid the fight against money laundering. The Financial Stability Board published an excellent report on 1 November about the impact of artificial intelligence that identified other ways it can help. Examples include AI-driven anti-impersonation checks that evaluate whether photos in different identity documents match, and using machine learning to identify customers that may pose a higher risk and so warrant, say, a deeper probe into the sources of their wealth.
I am focusing on transaction monitoring, but these other applications also hold promise.
The use of machine learning techniques does raise some questions.
- How can regulators become comfortable these systems are effective: if even the machine’s creators cannot know the reasons why the machine has made its recommendations, how can a regulator? The FSB explored this question in their report, asking how the lack of 'interpretability' and 'auditability' might pose dangers.
- Should machine learning complement existing transaction monitoring systems, or replace certain parts of the process, or even replace them entirely?
- What decisions are left for the humans?
Ultimately, we are chiefly concerned about whether these systems are effective and spot the needles in the haystack – the suspicious activities. They should primarily be judged on their performance outcomes. Sometimes a firm may need to carefully explore the trade-off between interpretability and performance when choosing what systems to use. What principles should the firm be using in making this choice?
The FSB did not provide an answer to this, and neither will I.
If regulators were to insist on a window into the machine’s inner workings, then this would, in effect, be a regulatory prohibition of the use of the more free form varieties of artificial intelligence where such a window is not possible. But what is it reasonable and proportionate for us to ask for? What we do expect to see is new technology implemented in a way you would any other – testing, governance and proper management. We as regulators clearly need to think more on this. We are encouraged that many firms out there are starting to develop their own code of ethics around data science; encouraging responsible innovation.
We are also often asked if we are happy for older more-traditional transaction monitoring systems to be decommissioned if they prove to be less effective. It would clearly be wasteful for parallel systems to be running beyond the time necessary to form a robust judgement about their relative usefulness. We are not expecting each system to flag everything; some approaches will flag certain transactions that others will not, but that is acceptable if, overall, one approach can be shown to be producing better quality alerts.
How should machine learning sit alongside human decision-making? We see it as complementing, not replacing, human judgement.
If a firm were trialling a new approach to overseeing its transactions, and comparing it to an older system, what other factors would they consider? The cost of achieving each actionable piece of intelligence could be an important consideration when comparing different approaches; the resources applied to tackling financial crime are finite, and it should go without saying it is desirable to use them efficiently.
How should machine learning sit alongside human decision-making? We see it as complementing, not replacing, human judgement. A feedback process is crucial to improving overall performance over time, with, for example, knowledge of which predictions were false positives (or false negatives) being used to continuously refine the model.
The machines can direct the humans to the cases of most interest. But the software will deal in probabilities, not absolutes, and a person will need to make the final decision about whether intelligence is passed to the authorities. People also need to be testing the machine and governing it.
So we agree that there are big potential benefits from the use of machine learning to tackle money laundering. But what are the limitations?
The effectiveness of a new technology always depends on its context. To illustrate this, consider a previous generation of data analysis technology. The statistical methods underpinning banks’ credit decisions have long been heavily computerised. These systems are also key to a lender’s viability as a going concern, so prudential supervisors have long taken an interest in how they work. But an understanding of the technology alone will not do: human factors can undermine the most sophisticated attempts to model and predict people’s behaviour. For example, a bank’s credit model that is able to reliably predict loan recoveries can be instantly thrown awry by a change in bankruptcy law. Meanwhile, imagine a situation where a lender’s heavily-incentivised sales staff begin to game the system, and coach uncreditworthy customers how to be granted a loan. Cases like this were particularly prevalent in the years leading to the financial crisis, and meant a worrying share of some lenders’ credit decisions were based on dubious data. Many of the borrowers fell into arrears, with all the hardship this entailed, while the lender’s data team would struggle to work out how much of its information was reliable and capable of informing future lending decisions.
Are there similar non-technological challenges facing the use of machine learning in anti-money laundering? Two spring to mind.
First, data quality can be patchy. A learning system depends on feedback, but banks often complain they get very little information back from the police after filing a suspicious activity report. This lessens their ability to train the machines to spot the cases of most concern. But progress is being made; the creation, for example, of the Joint Money Laundering Intelligence Taskforce (a group composed of several banks and law enforcement agencies), has allowed police and banks to cooperate better and may provide new fodder for the machines to chew on.
Second, an individual institution will only see a limited part of the picture. The part of a transaction they handle is just one piece of a jigsaw, but there are limitations to the legal scope for banks to share information with each other about a customer to determine whether a suspicion is truly justified. The recent Criminal Finances Act did create a clearer legal framework around this information sharing. Greater sharing of information between banks could do as much as anything else to improve the quality of the intelligence they are able to provide to the authorities.
Conclusion
In many ways, the questions I am raising here are not new. Financial firms’ handling of big data has been of interest to regulators for a long time. For decades, financial firms have been using large datasets, computers and statistical science to predict losses arising from fraud, insurance claims and credit defaults. Regulators have had to become comfortable with each new wave of technology; the risks have not always been identified and contained.
Artificial intelligence has the capability to greatly amplify the effectiveness of the machine’s human counterparts, but it will be a constant work in progress
Artificial intelligence has the capability to greatly amplify the effectiveness of the machine’s human counterparts, but it will be a constant work in progress. Any bank hoping for a black box in the corner that will sniff out the launderers will be disappointed, but the technology has the capability to better achieve what we all want: keeping finance clean.