Speech by Rob Gruppetta, Head of the Financial Crime Department, delivered at Chatham House.
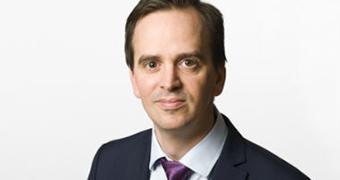
Speaker: Rob Gruppetta, Head of the Financial Crime Department
Event: Illicit Financial Flows 2018, Chatham House, London
Delivered: 19 November 2018
Note: this is the speech as drafted and may differ from the delivered version
Highlights
- We take a measured approach in keeping pace with innovation to help us combat financial crime, which remains one of our most important priorities.
- We recently published aggregated results from our first annual financial data return. For the first time, this gives a collective industry view of the financial crime risks that criminals pose to society and how UK firms are responding.
- Data and algorithms are improving our supervisory approach by helping to focus our efforts on where we think the risk is greatest.
Introduction
Tackling financial crime and money laundering remains one of our most important priorities. Money laundering can harm society by enabling criminal activity, and undermine the integrity of the entire financial system. Our job is to make sure that safeguards meet the latest international standards to make the UK a hostile place for criminals. But it’s not always easy: criminals keep evolving to stay one step ahead using new, sophisticated technologies to get the upper hand. But we’re not sitting still either. We’re always looking for ways to help us do a better job, and we’re not afraid to use new technologies to turn the tables on criminals.
We’re always looking for ways to help us do a better job, and we’re not afraid to use new technologies to turn the tables on criminals.
Regulation and innovation
The story of the innovator proving a sceptical establishment wrong is an appealing one. Think of Sir Frank Whittle and his jet engine designs. In the late 1930s, industry and government offered Whittle very little support, but, 10 years later, nearly all jet engines in use were based on his prototypes.
Another story – with a very different outcome - is that of the HMS Captain, a new type of ship launched in 1870. It was the brainchild of Captain Coles, who invented a new type of naval gun and wanted a ship built around it. The Royal Navy were doubtful, and thought the radical new design looked top-heavy. But Coles campaigned for his idea, got the backing of public opinion and the Navy relented. However, Coles’ triumph was short lived. Just months after its launch, HMS Captain capsized, with the loss of nearly 500 lives including Coles himself. An inquiry found that the design was fundamentally unstable. The Navy’s doubts were tragically justified.
So innovation should not be embraced without scrutiny, and the current artificial intelligence (AI) boom is no exception, as Professor Michael Jordan, world-renowned AI researcher and Professor of Statistics and Computer Science at the University of California warned earlier this year. He said that, just as we built buildings and bridges before there was civil engineering, we’re currently building large, complex AI decision-making systems in the absence of an engineering discipline with sound design principles. And just as early buildings and bridges sometimes fell to the ground unexpectedly and with tragic consequences, many early AI systems are exposing serious flaws in how we’re thinking about AI. While the building blocks of AI have started to emerge, sound principles for putting them together haven’t been developed yet. So as a regulator, a degree of scepticism about innovations like AI is rational.
as a regulator, a degree of scepticism about innovations like AI is rational.
For every transformative innovation, there will be many that are dead ends or even dangerous, with the potential to cause significant harm. Be that as it may, the FCA is also a champion for innovation where it helps us make better decisions and deliver better outcomes for consumers. That’s why we were the first regulator in the world to offer a regulatory sandbox – a ‘safe space’ where firms can test innovative products and services in the real market, with real consumers. We have also run 5 TechSprints or hackathons, including one on anti-money laundering (AML) and financial crime earlier this year. This event brought together more than 600 attendees from over 100 firms in 16 countries to explore how innovative tools can shine the light on financial crime. So where does this leave us as a regulator who is keen to foster measured innovation, but also wary of fuelling new dangers? We face a difficult balancing act.
Machine learning and financial crime
When it comes to AI, the spotlight is squarely on machine learning: a sub-field of AI that combines ideas from statistics, computer science and many other disciplines to design algorithms that process data, make predictions and help us make better decisions. The rise of machine learning is largely driven by the availability of ever larger datasets and benchmarks, cheaper and faster hardware, and advances in algorithms and their user-friendly interfaces being made available online.
This last point is particularly important. In the early days of machine learning, complex software had to be implemented from scratch to apply machine learning algorithms. These days the process is more like assembling LEGO blocks where the main pieces of the algorithms have already been built by experts and are available for free as open source software. This allows software engineers and data scientists to apply machine learning algorithms relatively easily to tackle a vast array of problems in finance, healthcare and public policy. This has helped fuel growth and investment in the field over the last decade, and it’s showing no signs of slowing down.
financial crime doesn’t lend itself easily to statistical analysis – the rules of the game aren’t fixed, the goal posts keep moving
Unsurprisingly, machine learning has also been on our radar. But financial crime doesn’t lend itself easily to statistical analysis – the rules of the game aren’t fixed, the goal posts keep moving, perpetrators change, so do their motives and the methods they use to wreak havoc. Simply turning an algorithm loose without thinking isn’t a suitable approach to tackling highly complex, dynamic and uncertain problems in financial crime.
That’s not to say we can’t use algorithms and models alongside our existing approach to help us be more consistent and effective in targeting financial crime risks. Consider building a risk model using algorithms: using a set of risk factors and outcomes, we could come up with a kind of mathematical caricature of how the outcomes might have been generated, so we can make future predictions about them in a systematic way. For example, in a money laundering context, the risk factors could be a firm’s products, types of customers and countries it deals with, and the outcomes could be detected instances of money laundering. Unfortunately, it’s quite difficult to acquire robust figures on money laundering as industry-wide data is hard to come by, and criminals aren’t exactly in the habit of publicising their successes. Crimes like money laundering – a secret activity that is designed to convert illicit funds into seemingly legitimate gains – is particularly hard to measure.
Supervised supervision
To address this data gap, we introduced a financial crime data return in 2016 to get an industry-wide view on key financial crime risks that firms face. We use the data to help us target our supervisory resources on firms that are exposed to high inherent risk. For example, the amount of cross-border business a firm conducts with risky countries or the proportion of wealthy, politically-exposed clients it holds in its client base.
The returns are filed annually, which will allow us to chart risks and trends over time. We published a report on financial crime analysis last week summarising our findings from the first year of returns. This will provide a collective industry view of the financial crime landscape for the first time. We believe the report will allow firms and other regulators to benchmark their own views about financial crime risks against industry-wide views.
From our perspective, the data return has allowed us to be more consistent, effective and risk-based in our supervisory approach than ever before. We are moving away from a rule-based, prescriptive world to a more data-driven, predictive place where we are using data to help us objectively assess the inherent financial crime risk posed by firms. And we have already started experimenting with supervised learning models to supervise the way we supervise firms – ‘supervised supervision’, as we call it.
Basically, we combine the data return with other data we hold about firms to paint a detailed picture of what a good and bad firm looks like, and focus our attention on those that show up at the bad end of the spectrum. Just to give you a glimpse of what’s possible – post- Markets in Financial Instruments Directive (MIFID) II, our market data processor is processing 30 million transaction reports per day – that’s a transaction for every person in London, New York and Tokyo combined. Deploying machine learning algorithms over this data gives us the ability to detect things that were previously impractical, like suspicious activity across different markets and venues. In this way, we’re squeezing the space that criminals can operate in.
Of course, we’re mindful that making predictions based solely on machine learning algorithms can be misleading, so we take great care to ensure that these are overlaid with appropriate financial crime and sector expertise. We only ever use them as the first step in a rigorous, multi-layered risk assessment process to help us target the riskiest firms. Simply put, the algorithms improve, rather than replace, supervisory judgment. The results so far look promising: year on year, we have improved risk targeting in our AML supervision work by over 65%.
Conclusion
I opened with the tale of the capsized HMS Captain, and the Navy’s failed attempts to prevent this disaster happening. As a regulator, we want to avoid the navy’s fate. Widespread popularity for a new idea is no guarantee that it won’t capsize. At the same time, there is no question that AI shows great promise in the long term – it could transform our industry by providing easier access to financial services, lowering cross-border transaction costs and improving the diversity of the system.
So we face a difficult balancing act. We remain open-minded, but appropriately sceptical about how we keep pace with innovation. As regulators, we will always be technology-agnostic, and the core tenets of our job will always remain the same: maintaining the integrity of the marketplace and ensuring better outcomes for consumers by weeding bad actors out of the system. To help us do this better, we will remain vigilant and adopt innovation in a targeted, systematic and measured manner: we don’t want to be left behind, but we won’t overdo it either.